Making an AI to make beautiful Art using GPT-3, CLIP and the CC12M Dataset.
Making an AI to make beautiful Art using GPT-3, CLIP and the CC12M Dataset.
So, my best friend is very talented in drawing and making art in over all. So, last time we were talking about a competition he had. He had to make two drawings for the topic utopia and dystrophia. Then I suddenly thought lets make an AI for this and as result here we are.
I started doing some research and found out openAI had an model named DALL E that can images based on text. So, I read the research paper and found out it works with something called GPT-3 and CLIP.
GPT-3 is one of the most advanced language processing model ever made.
CLIP is one of the most advanced language to image synthesis model ever made
Now I had the basics on how I will Design my AI but I needed more reference and then while researching I found out about “rivers have wings” a very talented coder who makes amazing models for art genration. I saw her using the CC12M dataset and with that I had everything ready and my research was a success.
So, Let's get started.
This project was made on google colab so first enable GPU in your runtime.
Then check your GPU:
Now lets start installing the dependencies:
Lets download the diffusion model:
Now for the imports:
Getting the data files of the diffusion model:
Lets start loading the models now:
Now lets take the inputs from the user:
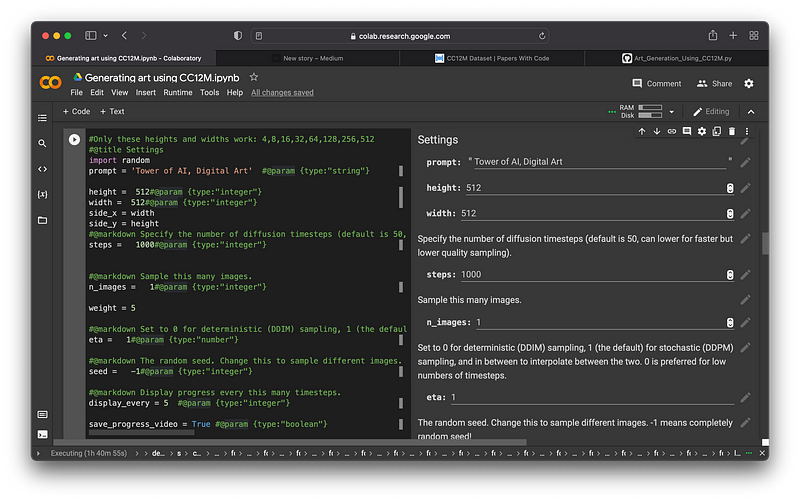
Now the full setup is ready now lets run the generator:
Lets add some markdown to see the generated video on Colab:
Now the code is done!!!
Let's generate some art!
First enter the parameters:
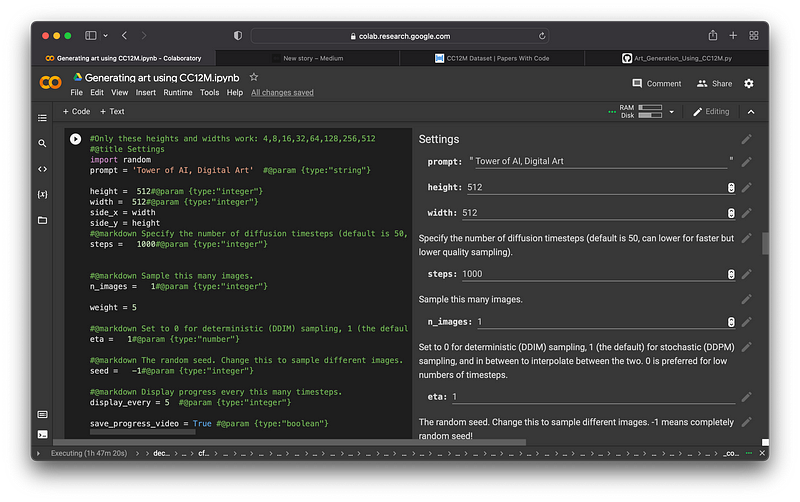
Now lets wait for the generation to complete:
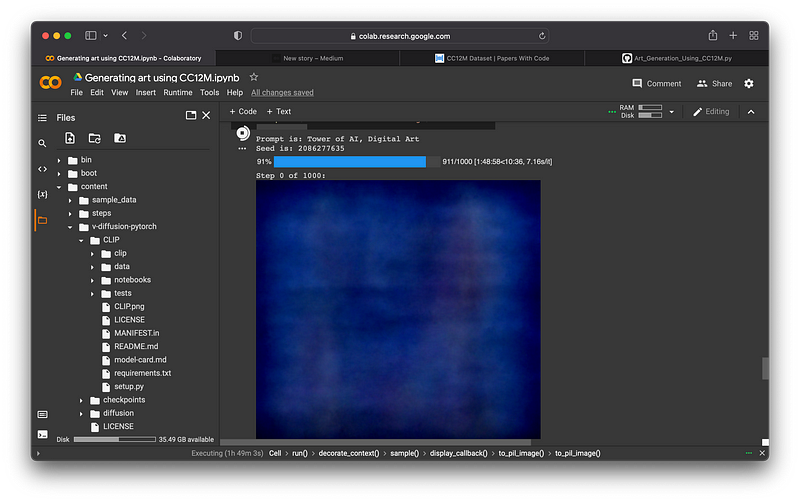
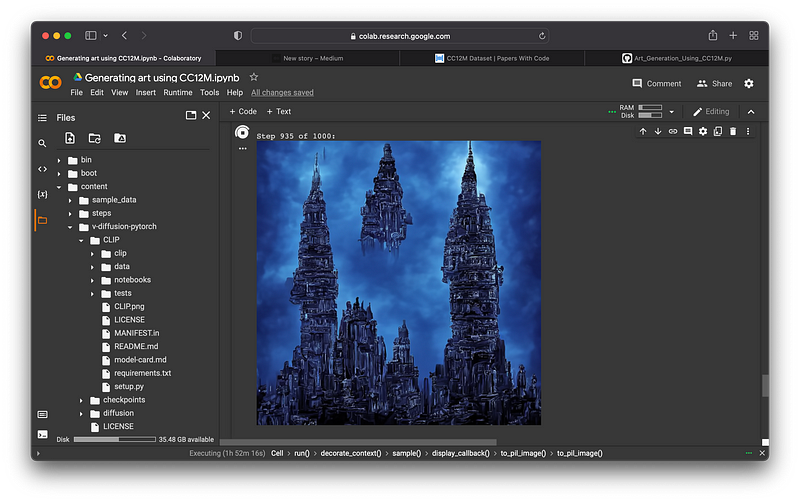
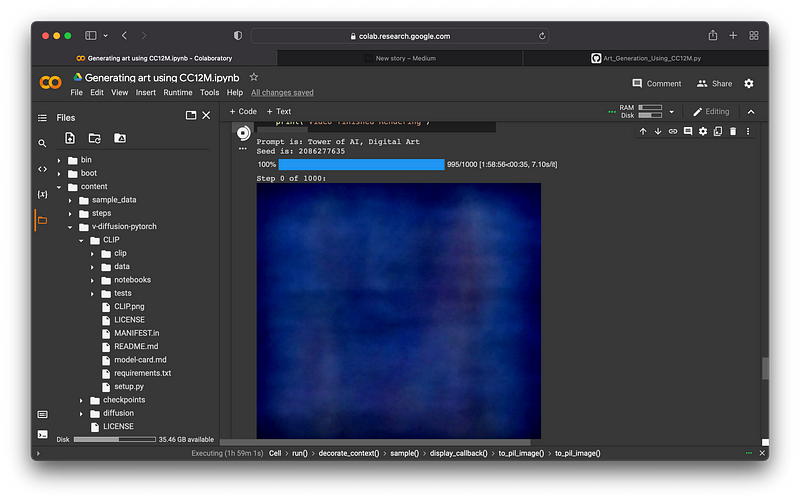
Ladies and Gentlemen I present to you “The Tower Of AI”
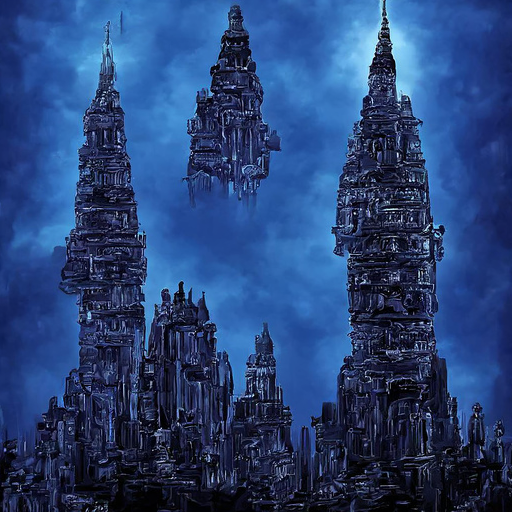
Its so beautiful 🤩
Lets see the video of it generating:
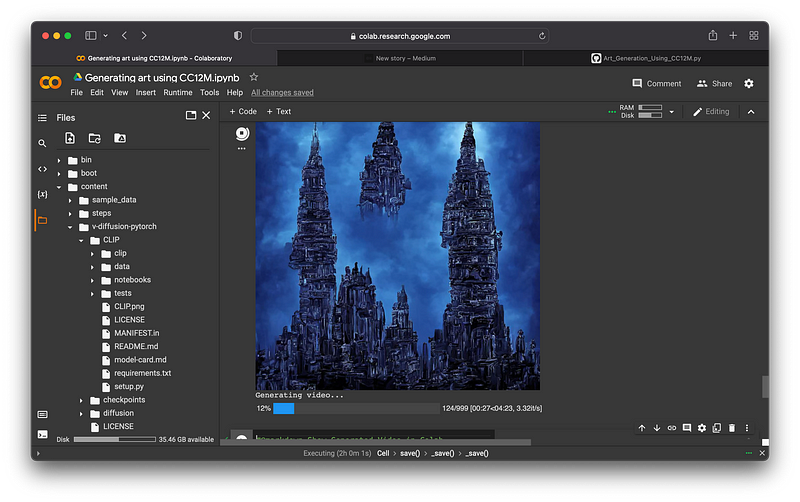
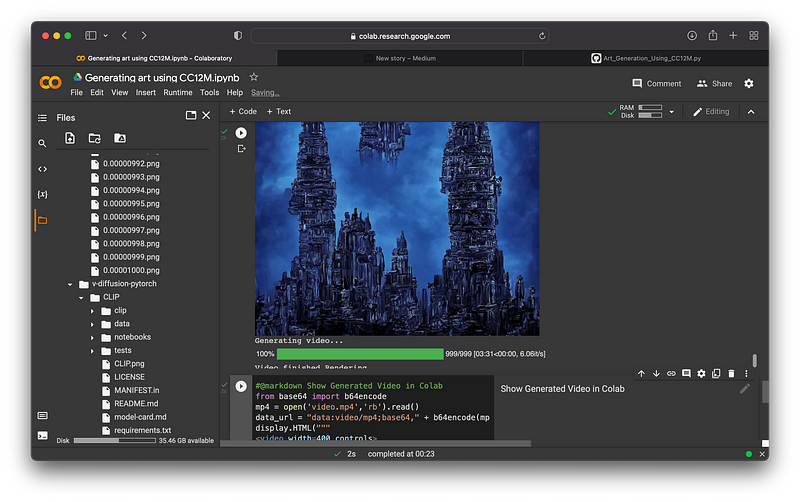
Lets see it:
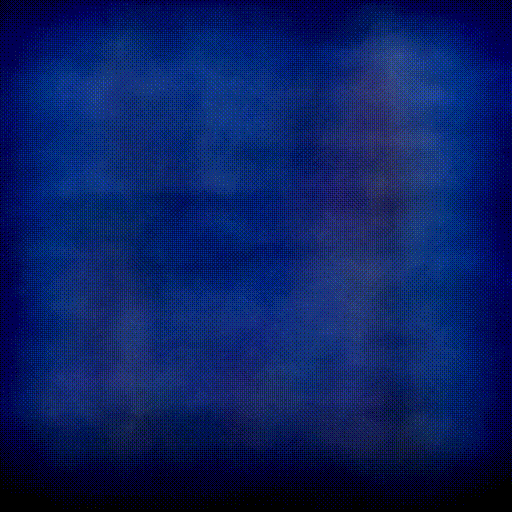
Lets see more Art:
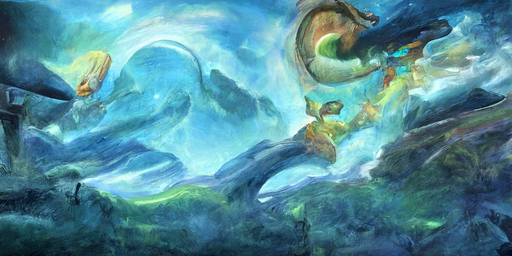
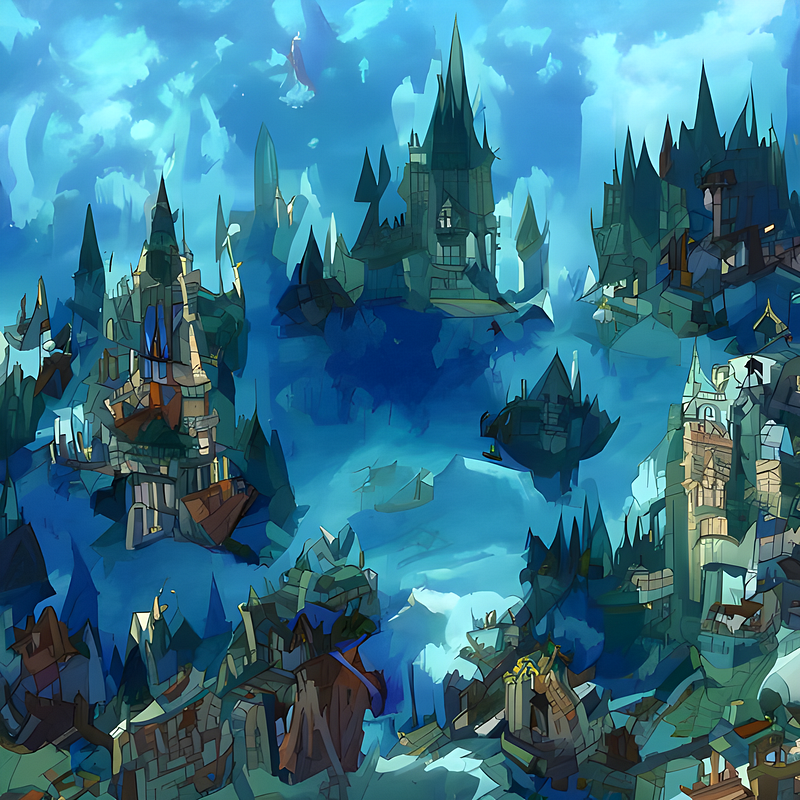
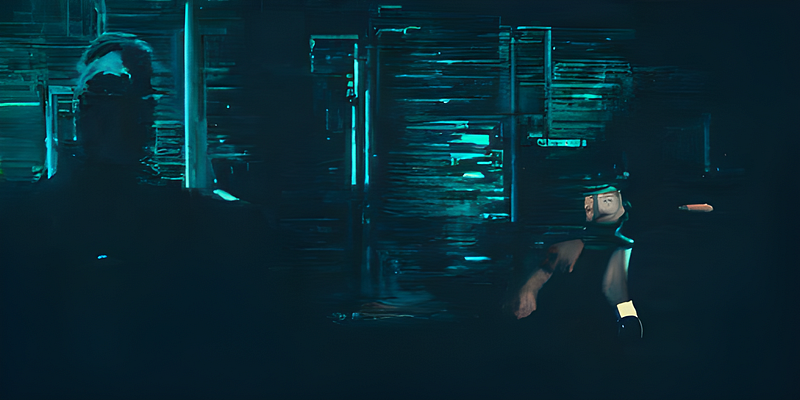
Well that concludes my AI is better than me at drawing and I am super happy about it. Hehe 😁
If you like this project a follow on medium is appreciated 😁.
If you wish to buy any of the four featured Art’s there NFT links are below each photo just click there name they are available for just $25.74 or 0.01 eth. It will help me out for my college funds and help make better articles.
Check out my best friends Art (now available as a NFT) that inspired this whole article: Here
I made them NFTs, go check it out: Here
Made an AI to make Artistic Animations check it out too:
So, two weeks ago I decided to make an AI to make Art and it was working beautifully then I was like why not make one…medium.com
For the Colab Notebook go here:
For more stuff check out my github:
You can't perform that action at this time. You signed in with another tab or window. You signed out in another tab or…github.com
For my day to day AIML research Updates follow me on twitter:
Thanks for reading😁, See ya guys next week 👋🏼.